Radiation Therapy
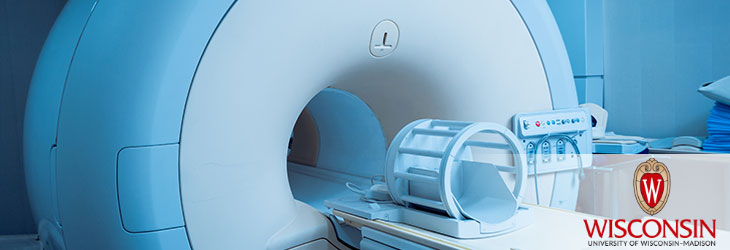
Image Autosegmentation Improves Treatment Planning
WARF: P130372US01
Inventors: Paulina Galavis, James Holden, Bhudatt Paliwal, Robert Jeraj
The Wisconsin Alumni Research Foundation (WARF) is seeking commercial partners interested in developing a method for processing and segmenting medical images that requires little or no user intervention and is parameter free.
Overview
Medical images are heavily relied upon to help plan radiation treatment and many other procedures. Not surprisingly, the success of any plan depends on the detail and accuracy of these images.
Imaging tools like PET (positron emission tomography) and SPECT (single photon emission tomography) can provide extensive physiological and biological information. PET, in particular, is used to identify and target tumors for irradiation. PET images are highly useful when tumors are hard to define by other imaging means, or when tumor boundaries are not easily distinguishable from surrounding tissue.
To properly define a target, images are divided into multiple parts in a process called segmentation. Properly segmenting images can be difficult given the inherent uncertainties of PET images. These uncertainties include physical as well as technical variables (e.g., blood glucose levels, motion, incorrect clock synchronization, etc.).
Imaging tools like PET (positron emission tomography) and SPECT (single photon emission tomography) can provide extensive physiological and biological information. PET, in particular, is used to identify and target tumors for irradiation. PET images are highly useful when tumors are hard to define by other imaging means, or when tumor boundaries are not easily distinguishable from surrounding tissue.
To properly define a target, images are divided into multiple parts in a process called segmentation. Properly segmenting images can be difficult given the inherent uncertainties of PET images. These uncertainties include physical as well as technical variables (e.g., blood glucose levels, motion, incorrect clock synchronization, etc.).
The Invention
UW–Madison researchers have developed a parameter-free, automatic image segmentation method that overcomes user variations.
To define a target for radiotherapy, for example, the process involves three stages. First, multiple highly discriminate texture feature images are generated. Then, a parameter-free NCRG (non-connected region-growing) is generated to determine the desired segmentation for each image that yields multiple regions of interest. Finally, the tumor region is determined using a synthesis and analysis algorithm.
The new method is called a gradient assisted non-connected automatic region (GANAR) analysis.
To define a target for radiotherapy, for example, the process involves three stages. First, multiple highly discriminate texture feature images are generated. Then, a parameter-free NCRG (non-connected region-growing) is generated to determine the desired segmentation for each image that yields multiple regions of interest. Finally, the tumor region is determined using a synthesis and analysis algorithm.
The new method is called a gradient assisted non-connected automatic region (GANAR) analysis.
Applications
- Medical image segmentation for treatment planning and assessment
Key Benefits
- Requires little or no user intervention
- Parameter free
- No need for system-dependent optimization
- Less sensitive to acquisition mode and reconstruction parameters
- More robust than other methods under varying reconstruction parameters
- Applicable to any lesion site
Stage of Development
In a study using PET-based simulated lesions, GANAR successfully dealt with irregular lesion shapes and variable activity concentrations. It performed better than threshold-based, gradient-based and region-growing segmentation methods. Results are valid across different tumor sites, such as lung, epiglottis, adrenal gland, esophagus and metastasis.
Additional Information
For More Information About the Inventors
Related Technologies
Tech Fields
For current licensing status, please contact Jeanine Burmania at [javascript protected email address] or 608-960-9846